Early CAREER Award will help researchers tap goldmine of hidden data
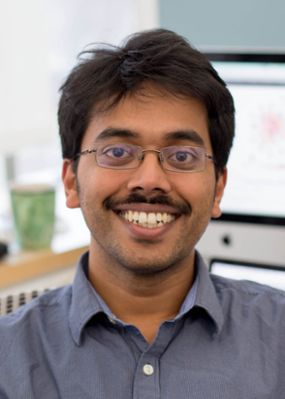
Michigan State University computational biologist Arjun Krishnan is the recipient of a 5-year, $704,889 National Science Foundation (NSF) Early CAREER Award to develop machine learning approaches that will automatically annotate publicly available samples from human and major animal models on a massive scale. His efforts will allow researchers to seamlessly search and re-analyze immense reserves of untapped omics data for advances in biology and human health.
Since the beginning of the omics field around 25 years ago, nearly every genomic, proteomic, transcriptomic and metabolomic data point has been stored in large, open access databases—a goldmine of data just waiting to be discovered.
“The most common types of data in humans and five major model organisms amounts to about 2 million samples that would be invaluable for scientists if they could take specific parts of the data relevant to their problem of interest and re-analyze them,” said Krishnan, assistant professor in the Department of Computational Mathematics, Science and Engineering, with a joint appointment in the Department of Biochemistry and Molecular Biology. “The data from all these samples are out there, but it is super hard to find the samples of interest.”
“I wanted to see if we could help others take advantage of the data the way we take advantage of it, that meant making the data searchable and reusable,” he added.
Krishnan and his research group will need to develop two new arms of machine learning to overcome two major obstacles to accessing the data. The first challenge: ambiguities inherent in human language.
“You want sample descriptions to be in a form that computers can read, quickly search and retrieve the right stuff, but it’s complicated by the fact that biologists use unstructured natural language meant for other scientists to read and further refer to the same attribute of samples using different words,” Krishnan explained. “For example, some people say heart attack and some people myocardial infraction, but they are referring to the same thing.”
Second, Krishnan explained that a lot of information about the original samples were not even recorded if not relevant to the original experiment. This includes invaluable details for future research like tissue of origin, developmental stage and physiological traits. But Krishnan is excited by the fact that the inherent nature of omics data means these details are not lost.
“It turns out that many attributes about the samples can be predicted from the molecular data measured from the samples,” Krishnan said. “For instance, if we know the activity of genes in genomes in a particular sample, we can predict what part of the body that sample came from, even if this information is completely missing from the description.”
Combining these two arms of machine learning will exponentially increase the scope of the project to systematically annotate 2 million samples, but machines aren’t the only ones who will be learning new skills when the grant starts this May.
Krishnan is using his Early CAREER Award to help students find what he calls the “hidden curriculum” of bioinformatics by developing online curriculum and in-person workshops that introduce undergraduates to abstract skills they would gain when working in a lab. Skills like how to set up a computational problem, how to manage data and code, how to communicate results and how to grow their professional network.
“My goal is to formalize and disseminate this kind of hidden curriculum as openly and widely as possible because it is the social capital that is often a big barrier to inclusivity and diversity,” said Krishnan.
He will do it with the help of R-Ladies East Lansing, a local group of 500+ computer programmers formed in 2018 to create a safe space for learning in R as a programing language and data science. Krishnan has been the faculty advisor since it began.
“The reason we are able to do this project is the people in my lab,” Krishnan emphasized. “They are extremely talented scientists who are also kind, supportive and love to give time to R-Ladies. I am most excited to continue to work with these scientists.”
Krishnan would be the first to admit that training machines in natural language processing is still unfamiliar bioinformatics territory, but the prospect of discovery was too exciting, and they had to try. The NSF agreed that developing a way to sift out valuable nuggets of data had significant future applications worth its weight in gold.
“Describing data better and better improves the effectiveness of the data analysis,” said Krishnan, who is bringing the full force of his lab’s expertise in algorithms to analyze text data. “We are starting with text descriptions of biological samples in this project, but there is still so much data out there related to biology and health in records and academic papers, so much knowledge hidden away.”
Krishnan is also the recipient of the prestigious Maximizing Investigators' Research Award from the National Institute of General Medical Sciences, National Institutes of Health. This award, which he received in 2018, supports the other major research mission of his group developing algorithms to understand the genetic and molecular basis of complex diseases.
Banner image: The machine learning approaches developed by the Krishnan Lab will automatically annotate publicly available samples from human and major animal models on a massive scale, enabling researchers to seamlessly discover relevant published data for use in further analysis. Credit: Arjun Krishnan