Innovative method develops more accurate corn yield predictions
Yield predictions are of great importance, from national and international food supply chains to the individual grower. In addition to ensuring food security, highly consequential financial decisions are made based on this information. Growers must decide how much fertilizer and other inputs to apply to their fields, for example, an area in which costs have soared for numerous reasons, including climate change and global conflict.
Newly published Michigan State University research shows that incorporating in-season water deficit information into remote sensing-based crop models drastically improves corn yield predictions.
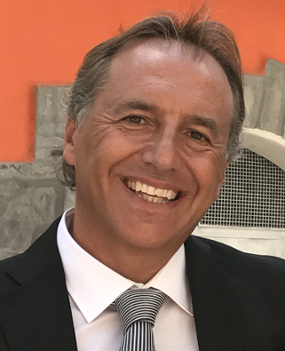
The study, led by Bruno Basso, an MSU Foundation Professor in the Department of Earth and Environmental Sciences in the College of Natural Science and a W.K. Kellogg Biological Station faculty member, was recently published in Remote Sensing of Environment, a leading journal in the field. Guanyuan Shuai, a graduate student in Basso’s lab, also participated in the study.
“An accurate knowledge of yield predictions before the end of the season is of paramount importance for grain prices, which affects profitability for farmers, as well as commodity traders and food companies,” said Basso, who also holds a joint appointment in the Department of Plant, Soil and Microbial Sciences in the College of Agriculture and Natural Resources.
Historical weather forecasts and crop yields from a given location are often used to predict the next season’s performance, but there are flaws in this methodology. This can be unsettling when growers are searching for as much certainty as possible.
“Farmers can now receive high-resolution weather information on their tablets and smartphones, but the U.S. Department of Agriculture yield outlooks are at the county level, so farmers can’t really use them to forecast yields on their fields,” Basso said. “What farmers need is knowledge to better manage and predict yields at the field and subfield levels. We know that circumstances vary within a field, even a small one of a few acres, let alone operations that include thousands of acres.
“It’s important that farmers have confidence in the data they use to make decisions, and we’re trying to help them improve that decision-making process at the right scale. Farmers are interested in profitability, which is also linked to environmental sustainability.”
For the project, Basso and Shuai evaluated 352 fields of varying sizes in Michigan, Indiana, Illinois and Iowa. The team collected climate and soil data, in addition to more than 2,500 yield maps over several years — 2006 to 2019 — directly from farmers.
They obtained high-resolution images from the private company Planet, the European Space Agency and NASA, along with digital elevation models from the U.S. Geological Survey dataset.
The images were used in part to calculate the green chlorophyll vegetation index, an indication of plant vigor that measures the chlorophyll content in leaves based on infrared and near-infrared imagery.

Basso and Shuai then implemented the System Approach to Land Use Sustainability (SALUS) program, which models crop, nutrient, soil and water conditions each day over many years using different management techniques. SALUS provided the daily crop drought index (CDI), designed to highlight the effect in-season water shortages has on crops.
“We found that the inclusion of the CDI substantially improved the accuracy of in-season predictions,” Basso said. “We showed that the greatest improvements in predictions were observed in the driest year, 2012. We also showed that the subfield spatial variations of corn yield are better captured with the inclusion of CDI for most fields.”
Basso believes that subfield-level analyses are a promising way to ensure accurate and precise predictions, and that decisions should be made based on real-time data rather than historical guidelines alone. He said that while some technology is being adopted broadly, the pace at which this is occurring must be expedited.
“Even if you use remote sensing imagery on your fields, that alone isn’t enough,” Basso said. “Once the canopy of the field comes close together, which occurs a month after planting or so, remote sensing will only see the top layer of those leaves, as one big green layer. Two months later, it will see the same big green layer but won’t be able to capture that the leaves and the plant have grown significantly below the canopy.
“Our novel, integrated approach of coupling crop-modeled water stress with high-resolution images is trying to remove this limitation in remote sensing image analysis," he continued. "This approach provides more reliable and timely information for farmer cost savings and environmental protection.”
This research was supported in part by the U.S. Department of Agriculture’s National Institute of Food and Agriculture; the Great Lakes Bioenergy Research Center; the U.S. Department of Energy’s Office of Science, and the Biological and Environmental Research program.
Banner image: Yield predictions are of great importance, from national and international food supply chains to the individual grower. Newly published Michigan State University research led by ecosystems scientist Bruno Basso shows that incorporating in-season water deficit information into remote sensing-based crop models drastically improves corn yield predictions. Credit: shutterstock/Peter Garbet.